Generative AI has emerged as one of the most exciting advancements in artificial intelligence. By leveraging powerful machine learning algorithms, generative AI can create new content—whether it’s text, images, music, or even video—on its own. What was once a concept limited to science fiction is now transforming industries across the globe.
In this blog post, we’ll dive into what generative AI is, how it works, its key applications, and the potential challenges and opportunities it presents.
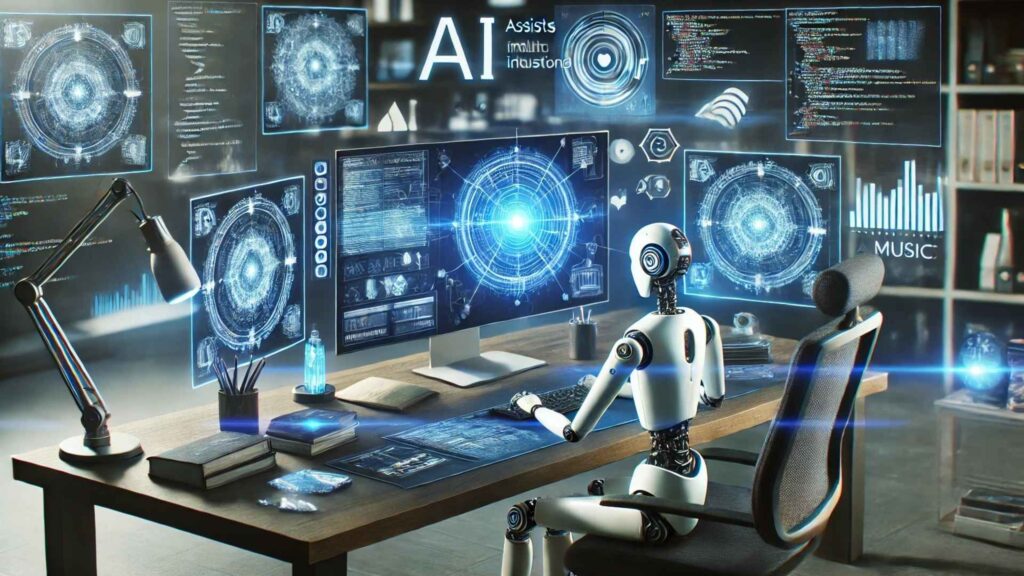
Table of Contents
What Is Generative AI?
Generative AI refers to a type of artificial intelligence that can generate new data by learning from existing data. Unlike traditional AI, which focuses on recognizing patterns or making decisions based on pre-existing information, generative AI creates entirely new content that didn’t exist before. It can produce human-like text, stunning visuals, deepfake videos, and even realistic-sounding music.
The most popular generative AI models include:
- GPT (Generative Pre-trained Transformer): Models like GPT-3 and GPT-4, developed by OpenAI, are widely used for text generation.
- DALL·E: Also developed by OpenAI, this model generates unique images based on textual descriptions.
- StyleGAN: Created by NVIDIA, this model generates highly realistic images, such as faces, landscapes, and artwork.
How Does Generative AI Work?
Generative AI typically uses deep learning techniques to create new content. At the heart of this process are neural networks—specifically, Generative Adversarial Networks (GANs) or Transformers.
1. Generative Adversarial Networks (GANs)
GANs consist of two neural networks: the generator and the discriminator. The generator creates new content, while the discriminator evaluates the content and determines if it’s real (i.e., resembling the original dataset) or fake. Over time, the generator improves its output until the discriminator can no longer tell the difference between real and generated content.
- Generator: Learns patterns in data and generates new examples.
- Discriminator: Determines if the output is authentic or artificial.
This iterative process leads to highly realistic outputs, such as fake images of people that are indistinguishable from real photographs.
2. Transformers
For text and language models, transformer architectures like GPT have become the gold standard. These models are trained on vast amounts of textual data, learning the relationships between words and phrases. When given a prompt, a transformer model can predict and generate sentences, paragraphs, or even entire articles that sound coherent and contextually appropriate.
Key Applications of Generative AI
Generative AI is finding applications in various fields, from content creation to product design. Here are some of the most impactful areas:
1. Content Creation (Text, Images, and Video)
Generative AI has been a game-changer in content generation. For writers, marketers, and designers, it automates tasks that previously took hours or days to complete.
- Text Generation: AI models like GPT-4 can write blog posts, social media updates, product descriptions, and more. This enables businesses to scale content marketing efforts without overloading human writers.
- Image and Graphic Design: Tools like DALL·E or MidJourney can generate visuals from text prompts. Whether you need a logo, illustration, or digital artwork, generative AI can create it from scratch, saving time and cost.
- Video Generation: AI-driven platforms can create short videos based on scripts or storyboards, and some tools even allow users to manipulate video content seamlessly. Deepfakes, while controversial, showcase the advanced capabilities of AI in mimicking realistic videos.
2. Personalized Marketing
Generative AI allows brands to create hyper-personalized marketing campaigns. By analyzing customer data and preferences, AI can generate individualized product recommendations, emails, and ad creatives tailored to each user. This enhances user engagement and can significantly improve conversion rates.
3. Product Design and Prototyping
In industries like fashion, architecture, and automotive design, generative AI is used to generate new product concepts. Designers input initial criteria, and the AI generates multiple iterations of the design, enabling rapid prototyping. This speeds up the design process and fosters more creative experimentation.
4. Music and Art Creation
Musicians and artists are increasingly using generative AI to push the boundaries of creativity. AI algorithms can compose original music tracks or assist in the creation of digital art. Platforms like Jukedeck and Amper Music are enabling non-musicians to compose unique pieces by leveraging AI’s generative capabilities.
5. AI in Gaming
Game developers are harnessing generative AI to create expansive, dynamic game environments. Rather than manually designing every detail, AI can automatically generate diverse and engaging landscapes, characters, and storylines. This reduces development time and offers gamers new experiences with each playthrough.
Challenges and Ethical Considerations
While generative AI offers immense potential, it also presents several challenges and ethical dilemmas:
1. Deepfakes and Misinformation
Generative AI’s ability to create realistic images and videos has raised concerns about deepfakes—AI-generated media that can be used to deceive or spread misinformation. This has far-reaching implications in areas like politics, media, and personal privacy.
2. Intellectual Property Concerns
As AI generates new content, questions arise around ownership. Who owns the rights to AI-generated music, artwork, or writing? Is it the creator of the algorithm, the user who inputs the prompt, or the AI itself? Current legal frameworks are still catching up with these developments.
3. Bias in AI
Like all machine learning models, generative AI can inherit biases from the datasets it is trained on. If the training data contains biases—whether gender, racial, or otherwise—the AI’s outputs may reflect these biases, leading to potentially harmful or unfair outcomes.
4. Job Displacement
The automation potential of generative AI is also raising concerns about job displacement, particularly in fields like content creation, design, and customer service. While AI can enhance productivity, there is fear that human jobs could be replaced by algorithms in certain areas.
Opportunities in Generative AI
Despite these challenges, generative AI offers vast opportunities across sectors:
1. Democratization of Creativity
Generative AI is making creativity accessible to everyone. You no longer need to be an expert designer, writer, or musician to create high-quality content. AI tools are empowering small businesses, startups, and individuals to produce professional-grade materials.
2. Enhanced Efficiency
By automating repetitive tasks, generative AI allows professionals to focus on more strategic, high-level activities. For instance, marketers can use AI to draft content while spending their time analyzing campaign performance and strategy.
3. Innovation in Healthcare
Generative AI can aid in drug discovery by simulating new molecular compounds, speeding up the process of identifying promising candidates for pharmaceutical treatments. It is also being used in medical imaging to enhance diagnostic accuracy.
Conclusion
Generative AI is reshaping the creative and business landscapes, enabling new possibilities and enhancing efficiency. From text and image generation to personalized marketing and beyond, the potential applications are endless. However, as with any disruptive technology, it’s essential to approach it with careful consideration of its ethical implications.
By embracing the opportunities and navigating the challenges responsibly, generative AI will continue to play a pivotal role in shaping the future of creativity, automation, and innovation.
“This Content Sponsored by Genreviews.Online
Genreviews.online is One of the Review Portal Site
Website Link: https://genreviews.online/
Sponsor Content: #genreviews.online, #genreviews, #productreviews, #bestreviews, #reviewportal”